What Are the Challenges in Data Science?
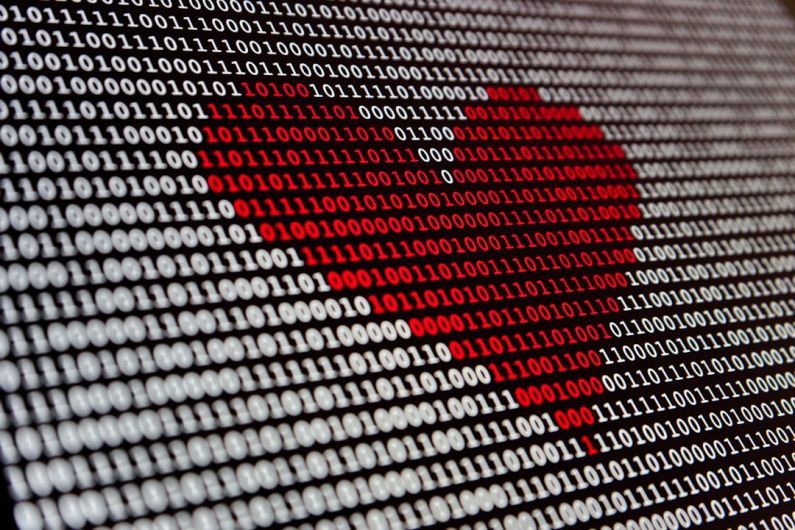
Data science has emerged as a crucial field in the modern world, with organizations across various industries relying on it to make data-driven decisions. However, navigating the realm of data science is not without its challenges. From data collection to model deployment, data scientists face a myriad of obstacles that can hinder the success of their projects. Understanding these challenges is essential for aspiring data scientists and organizations looking to leverage data science effectively. Let’s delve into some of the key challenges in data science.
**Data Quality and Quantity**
One of the fundamental challenges in data science is ensuring the quality and quantity of data. Data scientists often encounter incomplete, inconsistent, or biased data, which can lead to inaccurate insights and flawed models. Moreover, the sheer volume of data available today can be overwhelming, making it challenging to identify relevant information and extract meaningful patterns. Data quality issues can arise from various sources, such as human error, system glitches, or outdated data collection methods. Addressing these issues requires robust data cleaning techniques and a keen eye for detail to ensure that the data used for analysis is reliable and accurate.
**Data Privacy and Security**
With the increasing emphasis on data-driven decision-making, concerns surrounding data privacy and security have become more pronounced. Data scientists must navigate stringent regulations and ethical considerations to protect sensitive information and ensure compliance with data protection laws. Unauthorized access, data breaches, and misuse of data are significant risks that can have severe consequences for individuals and organizations. Implementing robust security measures, such as encryption and access controls, is essential to safeguard data privacy and maintain trust with stakeholders.
**Lack of Domain Knowledge**
Data science is a multidisciplinary field that requires expertise in statistics, programming, and domain-specific knowledge. One of the significant challenges for data scientists is bridging the gap between technical skills and domain expertise. Without a deep understanding of the industry or business context, data scientists may struggle to interpret results accurately or generate actionable insights. Collaboration with domain experts is crucial to contextualizing data analysis and deriving meaningful conclusions that align with organizational goals.
**Model Selection and Interpretability**
Selecting the right model for a data science project is a critical decision that can impact the accuracy and reliability of predictions. With a plethora of algorithms and techniques available, data scientists must evaluate the trade-offs between model complexity, interpretability, and performance. Additionally, ensuring the interpretability of models is essential for stakeholders to understand the reasoning behind predictions and build trust in the results. Balancing the need for accuracy with the need for transparency is a delicate task that requires careful consideration and expertise.
**Scalability and Deployment**
Scaling data science projects from small-scale experiments to production-ready systems can pose significant challenges for organizations. Deploying models into operational environments requires robust infrastructure, efficient workflows, and continuous monitoring to ensure optimal performance. Additionally, integrating data science solutions with existing systems and business processes can be complex and time-consuming. Data scientists need to collaborate closely with IT teams and stakeholders to streamline the deployment process and drive value from data science initiatives.
**Continuous Learning and Adaptation**
The field of data science is constantly evolving, with new technologies, tools, and techniques emerging at a rapid pace. Data scientists must stay abreast of the latest developments and trends to remain competitive and deliver innovative solutions. Continuous learning and adaptation are essential for honing skills, expanding knowledge, and tackling new challenges in data science. Embracing a growth mindset and cultivating a culture of learning within organizations can foster creativity, resilience, and excellence in data science practices.
**In Summary**
Navigating the complexities of data science requires a combination of technical expertise, domain knowledge, and strategic thinking. By addressing challenges such as data quality, privacy, domain knowledge, model selection, scalability, and continuous learning, data scientists can overcome obstacles and drive successful outcomes. Embracing these challenges as opportunities for growth and innovation can lead to impactful insights, informed decision-making, and transformative solutions in the dynamic landscape of data science.